PhD Thesis Defense | Claudia Borredon
On the dynamics of polymers and biomolecules through the use of machine learning algorithms
October 25, 11:00
CFM Auditorium
Candidate: Claudia Borredon
Supervisors: Gustavo A. Schwartz and Luis A. Miccio
DOWNLOAD FULL THESIS
The design and the development of new materials with desired physico-chemical properties often involves time-consuming and resource-intensive processes. To overcome these difficulties, machine learning and artificial neural networks (ANNs) have emerged as powerful tools within the framework of quantitative structure-property relationship (QSPR) models. In this thesis ANNs play a central role, as they are used to capture complex relationships between molecular structures and their properties. Among the numerous macroscopic properties of materials, this work is focused on the glass transition temperature (Tg), which holds significant academic and industrial relevance. Tg plays a crucial role in determining the mechanical, thermal, and processing characteristics of polymers and other glass forming materials. Despite its importance, understanding the physical mechanisms underlying the glass transition phenomenon remains challenging. In this work it was possible to obtain estimations on the dynamics of glass formers (molecular and polymers) starting from the prediction of the . With this, it is demonstrated that QSPR models offer a more time and cost-efficient alternative to experimental approaches, accelerating the screening and discovery of new materials with targeted glass transition behaviour.
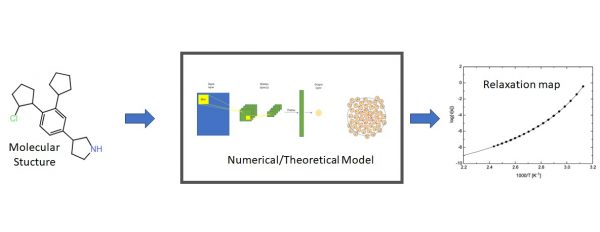
This image shows that starting from the molecular structure, with the use of artificial neural networks and theoretical models it is possible to obtain deeper knowledge about the molecular dynamics of the process.